
Intelligent dough mixing using AI
By Gary Tucker and Mike Adams - March 2020
For many, the term ‘artificial intelligence’ still conjures up futuristic and even dystopian imagery. Yet, what is fascinating about AI is that once it is in place and being used, it quickly becomes the accepted norm. Specialists in the field call it the Tesler Effect after a comment made by American computer scientist Larry Tesler way back in 1979 when seeking to define the technology: “AI is whatever hasn’t been done yet”.
We see this effect all around us. Once automation via technical marvel has been achieved, it becomes mundane. AI-type software drives speech and facial recognition as well as vision processing in photography, robotics and movie special effects nowadays without us giving it a second thought. In recent years, IT giants like Microsoft, Google and IBM have made AI technology cheaper and more available to software developers.
The challenge
The subject of bread making was one we thought as ideal for applying AI to model the process and ensure consistency in baking. Bread quality from an industrial bread processing plant needs to be consistent. However, there are numerous inputs and variables that interact to affect the crust and crumb quality. An Innovate UK grant was awarded to evaluate the feasibility of putting all this information together to demonstrate if it could work.
It is often said by bakers that the key processing operation that impacts most on the final product quality is dough mixing. The question we put to ourselves at the outset of our Innovate UK project was whether we could use AI technology to improve on bread dough mixing. The bread making process chosen for this was similar to that used industrially.
Britain has historically been a world-leader in bakery technology. In 1961, for example, our forerunner, the British Baking Industries Research Association invented the Chorleywood Bread Process (CBP). This was a new type of dough making process and it is now used all over the globe (in the UK, Australia, New Zealand, South Africa and India, where it makes up a high proportion of commercial bread production). It uses high shear mixing processes to fully develop dough in around three minutes, which does not leave much room for error.
Approximately 80% of Britain’s bread is now made in automated CBP plants. The numbers are staggering - an estimated 12 million loaves of bread and 2 million pizza bases are made in the UK every day (NABIM, 2019 Facts and Figures; Flour Milling in the UK). An automated bread line typically operates with two dough mixers feeding the line with dough at production rates of 8-10,000 kg/h. Getting the dough mixes right is critical to operational success. Poor quality dough has a major impact on each downstream processing stage and can cause equipment failure that generates waste. In particular, the softness and stickiness of dough are two important parameters for dough because it travels along conveyors and is compressed within dividers, rounders and moulders.
There are many factors that contribute towards the desired dough quality, and bakeries sometimes achieve poor dough quality during mixing. Getting dough mixes right is critical to operational success but difficult to achieve. This is because of unpredictable reactions between biology (yeast), chemistry (enzyme reactions, oxidation/reduction) and physics (water movement) that take place during bread processing.
The technology solution
AI solutions require data, and lots of it. Providing the AI model with plenty of bread processing data is essential. Consider, for example, the amount of human knowledge it takes to understand a topic such as crumb analysis, let alone the relationships between colour, dimensions, cell size, elongation and so on, as well as their optimal levels for every type of bread.
Our AI doesn’t learn or, indeed, learn to learn all this by itself. For it to work, real-life humans with specialist knowledge and, importantly, very clear aims need to teach it what is “good” and what is “bad” about all aspects of baking before it can make any salient judgements. While AI is about replacing tasks and improving upon them, this technology will always require some human decision-making.
This project highlighted two fundamental challenges in bringing AI to bear on any task.
- AI requires huge amounts of data, which is a challenge to collect
- The data must be input into the AI algorithm in a way that teaches it to appreciate its context
There were two sources of data used by the project, existing project data and new. We were able to access relevant sets of flour and baking data for a recent project in which the funding partners gave permission to use it. This enabled the project team to start managing the format for the data inputs to the AI software. One area where existing data was lacking was in the conditions that result in poor quality bread. This is unsurprising because it is rarely the objective of a baking trial to make poor bread. Consequently, we ran many mixing trials in which water temperature, mixing time and energy were changed so that the dough quality was deliberately poor, leading to lower quality bread than would normally be produced.
Table 1 presents some of the datasets that were collated, both objective and subjective, that had an impact of the dough mixing process. At the outset of the project it was not clear which parameters from the sets of data had the most significant impact on the dough and bread quality. For this reason the project team took the approach of inputting as much data as was thought relevant, as shown in Figure 1 by test results for a single bread baking test.
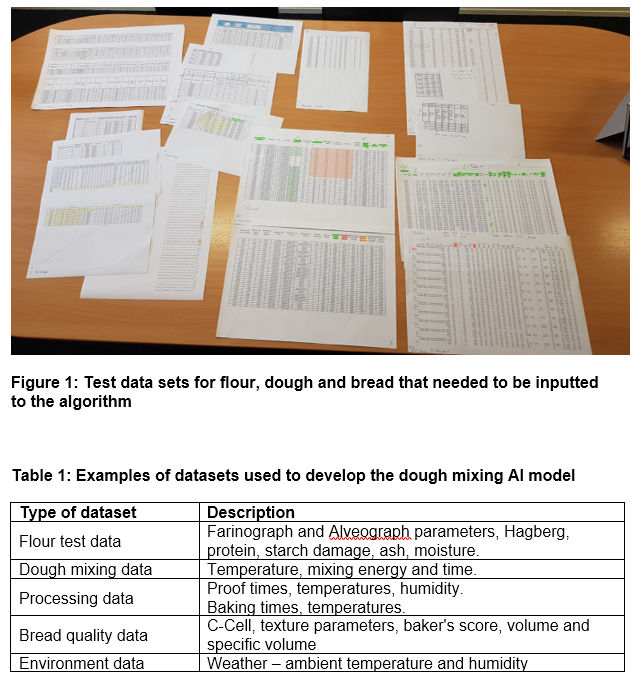
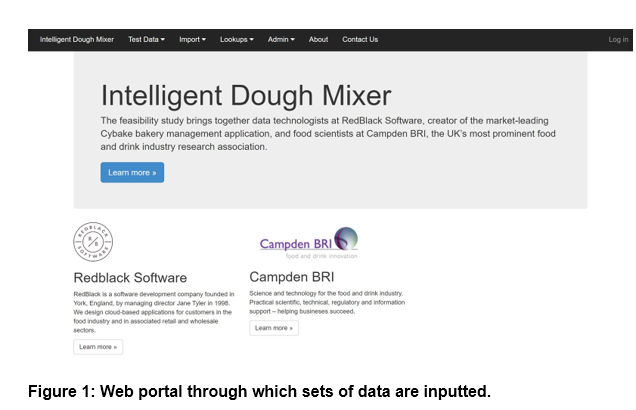
Results from the AI algorithm showed that it was possible to replicate predictions of bread quality from the set of input data. Changes in variables such as water temperature resulted in dough of different softness and stickiness, which in turn resulted in bread with changes to properties such as volume, colour, crumb structure and texture. The AI algorithm was able to predict these changes. This is a good starting position and now needs to be developed with an interactive system attached to a dough mixer.
Next stages
The completed Innovate UK project was a feasibility study that was able to demonstrate that the AI concept could be made to work. It now requires investment to take the algorithm and apply it to an actual dough mixer linked to the AI portal. The most likely way to de-risk this process is to apply for a further Innovate UK grant. Involvement of a UK mixer company, at least one bakery, and possibly an ingredient company would strengthen the partnership that already exists between Red Black and us at Campden BRI. The project team is looking to develop this case.
One of the discussion points was how to decide on the end point for the AI prediction – should we be focused on the measured dough or the finished bread properties? The premise at the outset was that controlling dough quality at the mixing stage was the single most important aspect of the process. Get this right and downstream processing will go smoothly. Get it wrong and changes need to be made to downstream settings to partially correct the situation. The latter tends to spiral out of control when dough of different quality goes through the system. By controlling mixing more accurately it should not be necessary to adjust moulder, prover and oven settings.
Thus, there is a strong argument that dough measurement after mixing is the correct way to implement an AI system. However, this is not as simple as it might seem. Measurements for dough softness and stickiness are not easy to take. There are no standard rheological or textural methods as with liquid or powder foods, or with baked products. This requires further development and could be part of the next stage project.
Conclusions
There are other examples of AI in the baking sector. Germany’s PreciBake specialises in AI systems that are virtually bolted on to ovens to automate their settings. In retail, Japan’s Brain Corps provides BakeryScan, a system that instantly scans and calculates prices for unpackaged breads, thus solving one of the biggest bugbears of self-service checkouts. Nearer to home, London’s catsAi is making a name for itself with AI-driven demand forecasting for bakeries.
The project on intelligent dough mixing is just the beginning. There are bound to be many ways in which AI weaves its way into the lives of bakers over the next decade that are still unforeseen. There may not be too much fanfare about all of this, but blink and you might miss it. In true Tesler effect-style, AI will already be quietly working away in the background at some mundane task without us ever even knowing.
We’re continually examining innovative technologies. How can fat, sugar and calories be reduced in bakery products? What are the novel technologies that can reduce waste during processing?
This article was first published in Baking Europe.